Financial forecasting is a crucial practice in both personal and corporate finance. It involves predicting future financial outcomes based on historical data, economic conditions, and various assumptions. Accurate financial forecasting helps businesses, investors, and individuals make informed decisions, plan for growth, manage cash flow, and mitigate risks. Whether you’re a startup planning its growth trajectory or an investor seeking to predict market trends, understanding the key methods used in financial forecasting is essential for making well-informed, data-driven decisions.
Financial forecasting provides a roadmap for budgeting, long-term planning, and strategic decision-making. It allows organizations to predict future sales, revenue, expenses, profits, and even potential risks. Given the increasing complexity of financial markets and business environments, financial forecasting has become a cornerstone of corporate strategy. In this article, we will explore the key methods used in financial forecasting, their applications, and how they can help organizations and individuals make better financial decisions.
Key Takeaways
- Financial forecasting involves predicting future financial outcomes using historical data, statistical models, and expert insights.
- Common forecasting methods include time series analysis, regression analysis, econometric models, expert judgment, and market research.
- Each method has its strengths and applications depending on the available data and the specific forecasting needs.
- Accurate forecasting helps businesses plan, allocate resources, mitigate risks, and make informed decisions about investments, sales, and budgeting.
- Financial forecasting is critical for both long-term strategic planning and short-term operational decision-making.
The Importance of Financial Forecasting
Before diving into the specific methods used in financial forecasting, it’s important to understand why forecasting is vital to financial management and decision-making. Financial forecasting serves several key purposes:
- Strategic Planning: Forecasting helps businesses set clear objectives and develop strategies to meet them. This can include setting sales targets, budgeting for expenses, or determining capital requirements.
- Cash Flow Management: Accurate forecasts enable companies to anticipate periods of cash shortages or surpluses, helping them make decisions about borrowing, investment, or cost-cutting measures.
- Investment Decisions: Investors use financial forecasts to assess the future performance of a company, helping them make decisions about buying, selling, or holding stocks or bonds.
- Risk Mitigation: Forecasting helps identify potential risks in a business or investment. By recognizing potential negative scenarios in advance, companies can implement strategies to mitigate risks.
- Resource Allocation: Forecasting allows organizations to allocate resources effectively by anticipating future needs for labor, raw materials, capital, and infrastructure.
Now that we understand the importance of financial forecasting, let’s explore the methods commonly used in financial forecasting.
Key Methods in Financial Forecasting
There are various methods used in financial forecasting, each with its own set of strengths and limitations. The choice of method often depends on the type of business, the nature of the data available, the level of accuracy required, and the time horizon for the forecast. The most commonly used methods include quantitative approaches such as time series analysis, regression analysis, and econometric models, as well as qualitative methods like expert judgment and market research. Below, we’ll explore these methods in detail.
Time Series Analysis
Time series analysis is one of the most widely used methods for financial forecasting, especially in industries where historical data plays a significant role in predicting future trends. Time series analysis involves analyzing historical data points collected over regular intervals, such as daily, monthly, or quarterly, and using them to identify patterns, trends, and seasonal variations.
The key components of time series data include:
- Trend: The long-term movement in the data, such as consistent growth or decline.
- Seasonality: Regular fluctuations that occur at specific intervals, often due to external factors like holidays or weather patterns.
- Cyclic Patterns: Recurrent patterns that are not as predictable as seasonal changes but can be influenced by economic cycles or business cycles.
- Random Fluctuations: Unexpected, unpredictable variations that do not follow any discernible pattern.
In time series forecasting, historical data is plotted on a graph to detect patterns, and these patterns are then used to make predictions about future events. Various statistical techniques, such as moving averages, exponential smoothing, and autoregressive integrated moving average (ARIMA), are used to analyze the data and generate forecasts.
Applications of Time Series Analysis:
- Predicting sales based on past performance.
- Forecasting demand for products or services.
- Estimating future cash flows or earnings.
Regression Analysis
Regression analysis is another important method used in financial forecasting. Unlike time series analysis, which focuses on historical data, regression analysis seeks to understand the relationship between two or more variables. By identifying how one variable (the dependent variable) is influenced by others (independent variables), regression analysis can help forecast future outcomes.
The most common type of regression used in financial forecasting is linear regression, where the relationship between the dependent and independent variables is assumed to be linear. For example, a company may use regression analysis to predict future sales based on factors like advertising spending, economic conditions, and product pricing. The relationship is expressed as a linear equation, and the future value of the dependent variable is calculated by plugging in the values of the independent variables.
Applications of Regression Analysis:
- Estimating the impact of marketing campaigns on sales.
- Predicting future sales based on economic indicators such as GDP growth or consumer confidence.
- Forecasting expenses based on operational factors like production volume or staffing levels.
Econometric Models
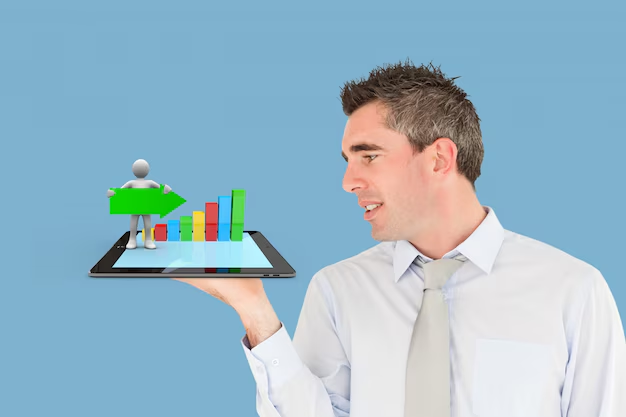
Econometric models are more sophisticated than basic regression analysis and involve using statistical techniques to quantify relationships between multiple economic variables. These models often incorporate data from various sources, including historical financial data, macroeconomic indicators, and industry-specific factors. Econometric models can handle complex relationships between variables and account for external factors such as government policies, interest rates, and inflation.
Econometric models typically require more advanced knowledge of statistics and economics and are used for long-term forecasts or when a detailed understanding of the underlying economic environment is required. These models are commonly used by large corporations, governments, and financial institutions to predict trends in areas such as GDP growth, inflation, and interest rates.
Applications of Econometric Models:
- Forecasting macroeconomic indicators such as inflation, interest rates, and unemployment.
- Predicting the effects of policy changes (e.g., tax rates, government spending) on the economy.
- Long-term financial forecasting for industries like energy, agriculture, and real estate.
Expert Judgment
While quantitative methods such as time series analysis and regression analysis are essential for data-driven forecasts, expert judgment is another valuable method for financial forecasting. This qualitative approach relies on the insights and experiences of industry experts, business leaders, or financial analysts to predict future outcomes.
Expert judgment is particularly useful in situations where data is limited, highly uncertain, or unavailable. It is also valuable when predicting events that are difficult to quantify, such as the potential success of a new product or the impact of disruptive technologies on a market.
Experts use their knowledge of industry trends, competitive dynamics, and macroeconomic conditions to make informed forecasts. These insights are often supplemented by market research, customer surveys, and focus groups to gather additional data points.
Applications of Expert Judgment:
- Forecasting demand for new or innovative products.
- Estimating the financial impact of uncertain or emerging market trends.
- Predicting the outcomes of political or regulatory changes.
Market Research and Surveys
Market research and surveys are often used in combination with other forecasting methods to gather insights directly from consumers, customers, and industry professionals. These methods provide valuable qualitative data, such as customer preferences, buying behavior, and market sentiment, which can be incorporated into financial forecasts.
Market research often involves surveys, focus groups, and in-depth interviews, while customer data analytics can help businesses understand their target audience better. This information is particularly useful for companies that are launching new products, entering new markets, or looking to expand their customer base.
Applications of Market Research and Surveys:
- Forecasting customer demand for new products or services.
- Understanding market trends and sentiment to predict future sales.
- Analyzing competitive dynamics and identifying potential opportunities for growth.
Moving Averages
The moving average method is one of the simplest and most commonly used forecasting techniques. It is a statistical calculation that smooths out fluctuations in time series data by averaging data points over a specific time period, such as 3, 6, or 12 months. This method is particularly useful for identifying trends and patterns over time, while filtering out short-term fluctuations.
There are different types of moving averages, including:
- Simple Moving Average (SMA): An unweighted average of data points over a specific period.
- Exponential Moving Average (EMA): A weighted average that gives more importance to recent data points, making it more sensitive to recent changes.
Applications of Moving Averages:
- Identifying long-term trends in stock prices.
- Forecasting demand or sales patterns based on historical data.
- Smoothing out short-term fluctuations in financial data to focus on long-term trends.
Also Read: How Can Financial Analysis Help In Making Better Investment Decisions?
Conclusion
Financial forecasting is a vital tool for businesses, investors, and individuals to make informed decisions, plan for the future, and manage financial risks. Whether using time series analysis, regression models, expert judgment, or market research, each method plays a unique role in predicting future financial outcomes. The key is to choose the right method based on the available data, the nature of the forecast, and the level of certainty required.
By integrating various forecasting methods, organizations can better anticipate market trends, allocate resources effectively, and mitigate financial risks. Financial forecasting, when done accurately, is an indispensable part of sound financial management.
Frequently Asked Questions(FAQs)
What is the best method for financial forecasting?
The best method depends on the type of data available, the level of accuracy needed, and the time horizon for the forecast. In practice, many companies use a combination of methods, such as time series analysis and expert judgment, to improve the reliability of their forecasts.
How far in advance can financial forecasting predict future performance?
The accuracy of financial forecasts diminishes as the forecast horizon extends. Short-term forecasts (1–2 years) are typically more accurate than long-term forecasts (5–10 years), especially when external variables such as market conditions or regulations are difficult to predict.
Can financial forecasting be used for personal finance?
Yes, financial forecasting is useful for personal finance as well. It can help individuals predict future income, expenses, savings, and investment returns, allowing for better financial planning and goal setting.
How do economic indicators influence financial forecasting?
Economic indicators such as GDP growth, inflation rates, and unemployment figures provide valuable insights into the overall health of the economy. These indicators are often incorporated into econometric models to predict future business conditions.
What is the role of expert judgment in financial forecasting?
Expert judgment is valuable when quantitative data is insufficient or uncertain. Experts draw on their knowledge and experience to provide insights into future trends, market behavior, and potential risks.
What are the limitations of financial forecasting?
Financial forecasting is subject to uncertainty and is often influenced by external factors that cannot be predicted accurately, such as changes in government policy, natural disasters, or technological disruptions. The accuracy of a forecast depends on the quality of the data and the assumptions used in the model.
Can financial forecasting help mitigate risks?
Yes, financial forecasting helps identify potential risks and prepare for them. By predicting future cash flow shortages, market downturns, or operational inefficiencies, businesses can implement strategies to reduce the likelihood or impact of these risks.